ARIMA Model - MissingDataError: exog contains inf or nans
19,972
There are some missing values in your dataset, you need to preprocess your data before passing it to the seasonal_decompose method.
indexedDataset = dataset.set_index(['Date'])
indexedDataset = indexedDataset.fillna(method='ffill')
You can also check other methods to fill your missing values from here
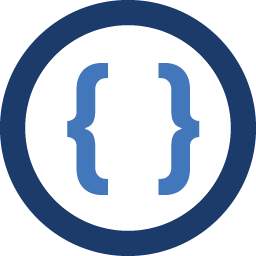
Author by
Admin
Updated on June 04, 2022Comments
-
Admin almost 2 years
I am trying to forecast few values using ARIMA Model. I get the following error. I have tried to remove the stationarity and other necessary conditions for the forecasting. Can someone point me out why this error is generated and how to fix this? Im new to Python. Thanks in advance.
Error completer error tree as follows.
MissingDataError Traceback (most recent call last) <ipython-input-7-35993c1e078a> in <module> 37 from statsmodels.tsa.stattools import adfuller 38 print("Results of Dickey-Fuller Test:") ---> 39 dftest = adfuller(indexedDataset["like"], autolag='AIC') 40 41 dfoutput = pd.Series(dftest[0:4],index=['Test Statistics','p-value', '#Lags Used','#Number of observations used']) ~\AppData\Local\Continuum\anaconda3\lib\site-packages\statsmodels\tsa\stattools.py in adfuller(x, maxlag, regression, autolag, store, regresults) 239 if not regresults: 240 icbest, bestlag = _autolag(OLS, xdshort, fullRHS, startlag, --> 241 maxlag, autolag) 242 else: 243 icbest, bestlag, alres = _autolag(OLS, xdshort, fullRHS, startlag, ~\AppData\Local\Continuum\anaconda3\lib\site- packages\statsmodels\tsa\stattools.py in _autolag(mod, endog, exog, startlag, maxlag, method, modargs, fitargs, regresults) 84 method = method.lower() 85 for lag in range(startlag, startlag + maxlag + 1): ---> 86 mod_instance = mod(endog, exog[:, :lag], *modargs) 87 results[lag] = mod_instance.fit() 88 ~\AppData\Local\Continuum\anaconda3\lib\site-packages\statsmodels\regression\linear_model.py in __init__(self, endog, exog, missing, hasconst, **kwargs) 815 **kwargs): 816 super(OLS, self).__init__(endog, exog, missing=missing, --> 817 hasconst=hasconst, **kwargs) 818 if "weights" in self._init_keys: 819 self._init_keys.remove("weights") ~\AppData\Local\Continuum\anaconda3\lib\site-packages\statsmodels\regression\linear_model.py in __init__(self, endog, exog, weights, missing, hasconst, **kwargs) 661 weights = weights.squeeze() 662 super(WLS, self).__init__(endog, exog, missing=missing, --> 663 weights=weights, hasconst=hasconst, **kwargs) 664 nobs = self.exog.shape[0] 665 weights = self.weights ~\AppData\Local\Continuum\anaconda3\lib\site-packages\statsmodels\regression\linear_model.py in __init__(self, endog, exog, **kwargs) 177 """ 178 def __init__(self, endog, exog, **kwargs): --> 179 super(RegressionModel, self).__init__(endog, exog, **kwargs) 180 self._data_attr.extend(['pinv_wexog', 'wendog', 'wexog', 'weights']) 181 ~\AppData\Local\Continuum\anaconda3\lib\site-packages\statsmodels\base\model.py in __init__(self, endog, exog, **kwargs) 210 211 def __init__(self, endog, exog=None, **kwargs): --> 212 super(LikelihoodModel, self).__init__(endog, exog, **kwargs) 213 self.initialize() 214 ~\AppData\Local\Continuum\anaconda3\lib\site-packages\statsmodels\base\model.py in __init__(self, endog, exog, **kwargs) 62 hasconst = kwargs.pop('hasconst', None) 63 self.data = self._handle_data(endog, exog, missing, hasconst, ---> 64 **kwargs) 65 self.k_constant = self.data.k_constant 66 self.exog = self.data.exog ~\AppData\Local\Continuum\anaconda3\lib\site-packages\statsmodels\base\model.py in _handle_data(self, endog, exog, missing, hasconst, **kwargs) 85 86 def _handle_data(self, endog, exog, missing, hasconst, **kwargs): ---> 87 data = handle_data(endog, exog, missing, hasconst, **kwargs) 88 # kwargs arrays could have changed, easier to just attach here 89 for key in kwargs: ~\AppData\Local\Continuum\anaconda3\lib\site-packages\statsmodels\base\data.py in handle_data(endog, exog, missing, hasconst, **kwargs) 631 klass = handle_data_class_factory(endog, exog) 632 return klass(endog, exog=exog, missing=missing, hasconst=hasconst, --> 633 **kwargs) ~\AppData\Local\Continuum\anaconda3\lib\site-packages\statsmodels\base\data.py in __init__(self, endog, exog, missing, hasconst, **kwargs) 77 78 # this has side-effects, attaches k_constant and const_idx ---> 79 self._handle_constant(hasconst) 80 self._check_integrity() 81 self._cache = resettable_cache() ~\AppData\Local\Continuum\anaconda3\lib\site-packages\statsmodels\base\data.py in _handle_constant(self, hasconst) 131 ptp_ = self.exog.ptp(axis=0) 132 if not np.isfinite(ptp_).all(): --> 133 raise MissingDataError('exog contains inf or nans') 134 const_idx = np.where(ptp_ == 0)[0].squeeze() 135 self.k_constant = const_idx.size MissingDataError: exog contains inf or nans
import numpy as np import pandas as pd import matplotlib.pylab as plt %matplotlib inline from matplotlib.pylab import rcParams rcParams['figure.figsize'] = 10, 6 dataset = pd.read_csv("data.csv") #Parse strings to datetime type dataset['Date'] = pd.to_datetime(dataset['Date'], infer_datetime_format=True) indexedDataset = dataset.set_index(['Date']) from datetime import datetime indexedDataset.tail(5) #plot graph plt.xlabel("Date") plt.ylabel("Number of Likes") plt.plot(indexedDataset) #Determining the rolling statistics rolmean = indexedDataset.rolling(window=12).mean() rolstd = indexedDataset.rolling(window=12).std() print(rolmean, rolstd) #plot tolling statistics orig = plt.plot(indexedDataset, color="blue", label="original") mean = plt.plot(rolmean, color="red", label="Rolling Mean") std = plt.plot(rolstd, color="black", label= "Rolling std") plt.legend(loc="best") plt.title=("Rolling Mean and Standard Deviation") #Perform Dickey-Fuller test from statsmodels.tsa.stattools import adfuller print("Results of Dickey-Fuller Test:") dftest = adfuller(indexedDataset["like"], autolag='AIC') dfoutput = pd.Series(dftest[0:4],index=['Test Statistics','p-value', '#Lags Used','#Number of observations used']) for key, value in dftest[4].items(): dfoutput['Critical Value (%s)' %key] = value print(dfoutput) #Estimating trend indexedDataset_logScale = np.log(indexedDataset) plt.plot(indexedDataset_logScale) movingAverage = indexedDataset_logScale.rolling(window=12).mean() movingSTD = indexedDataset_logScale.rolling(window=12).std() plt.plot(indexedDataset_logScale) plt.plot(movingAverage, color="red") datasetLogScaleMinusMovingAverage = indexedDataset_logScale - movingAverage datasetLogScaleMinusMovingAverage.head(12) #remove Nan Values datasetLogScaleMinusMovingAverage.dropna(inplace=True) datasetLogScaleMinusMovingAverage.head(10) from statsmodels.tsa.stattools import adfuller def test_stationarity(timeseries): #determing rolling statistics movingAverage = timeseries.rolling(window=12).mean() movingSTD = timeseries.rolling(window=12).std() #plot rolling statistics orig = plt.plot(timeseries, color='blue',label='Original') mean = plt.plot(movingAverage, color='red', label='Rolling Mean') std = plt.plot(movingSTD, color='black', label= 'Rolling std') plt.legend(loc='best') plt.title=("Rolling Mean and Standard Deviation") plt.show(block=False) #Perform Dickey-Fuller test: print('Results of Dickey-Fuller Test:') dftest = adfuller(indexedDataset["like"], autolag='AIC') dfoutput = pd.Series(dftest[0:4],index=['Test Statistics','p-value', '#Lags Used','#Number of observations used']) for key,value in dftest[4].items(): dfoutput['Critical Value (%s)'%key] = value print(dfoutput) test_stationarity(datasetLogScaleMinusMovingAverage) exponentialDecayWeightAverage = indexedDataset_logScale.ewm(halflife=12,min_periods=0,adjust=True).mean() plt.plot(indexedDataset_logScale) plt.plot(exponentialDecayWeightAverage, color='red') datasetLogScaleMinusMovingAverageExponentialDecayAverage = indexedDataset_logScale - exponentialDecayWeightAverage test_stationarity(datasetLogScaleMinusMovingAverageExponentialDecayAverage) datasetLogDiffShifting = indexedDataset_logScale - indexedDataset_logScale.shift() plt.plot(datasetLogDiffShifting) datasetLogDiffShifting.dropna(inplace=True) test_stationarity(datasetLogDiffShifting) from statsmodels.tsa.seasonal import seasonal_decompose decomposition = seasonal_decompose(indexedDataset_logScale) trend = decomposition.trend seasonal = decomposition.seasonal residual = decomposition.resid plt.subplot(411) plt.plot(indexedDataset_logScale, label='Original') plt.legend(loc='best') plt.subplot(412) plt.plot(trend, label='Trend') plt.legend(loc='best') plt.subplot(413) plt.plot(seasonal,label="Seasonality") plt.legend(loc='best') plt.subplot(414) plt.plot(residual, label='Residuals') plt.legend(loc='best') plt.tight_layout() decomposedLogData = residual decomposedLogData.dropna(inplace=True) test_stationarity(decomposedLogData) decomposedLogData = residual decomposedLogData.dropna(inplace=True) test_stationarity(decomposedLogData) #ACF and PACF plates from statsmodels.tsa.stattools import acf, pacf lag_acf = acf(datasetLogDiffShifting, nlags=20) lag_pacf = pacf(datasetLogDiffShifting, nlags=20, method="ols") #plot ACF plt.subplot(121) plt.plot(lag_acf) plt.axhline(y=0,linestyle='--',color='gray') plt.axhline(y=-1.96/np.sqrt(len(datasetLogDiffShifting)),linestyle='-- ',color='gray') plt.axhline(y=1.96/np.sqrt(len(datasetLogDiffShifting)),linestyle='-- ',color='gray') # plt.title("Autocorrelation Function") #Plot PACF plt.subplot(122) plt.plot(lag_pacf) plt.axhline(y=0,linestyle='--',color='gray') plt.axhline(y=-1.96/np.sqrt(len(datasetLogDiffShifting)),linestyle='--',color='gray') plt.axhline(y=1.96/np.sqrt(len(datasetLogDiffShifting)),linestyle='--',color='gray') # plt.title("Partial Autocorrelation Function") plt.tight_layout() from statsmodels.tsa.arima_model import ARIMA #AR MODEL model = ARIMA(indexedDataset_logScale, order=(2, 1, 2)) result_AR = model.fit(disp=-1) plt.plot(datasetLogDiffShifting) plt.plot(result_AR.fittedvalues, color='red') print('RSS: %.4f'% sum((result_AR.fittedvalues- datasetLogDiffShifting["like"])**2)) print('Plotting AR model') #MA MODEL model = ARIMA(indexedDataset_logScale, order=(2,1,2)) results_MA = model.fit(disp=-1) plt.plot(datasetLogDiffShifting) plt.plot(results_MA.fittedvalues, color='red') print('RSS: %.4f'% sum((results_MA.fittedvalues- datasetLogDiffShifting["like"])**2)) print('Plotting AR model') model = ARIMA(indexedDataset_logScale, order=(2,1,2)) results_ARIMA = model.fit(disp=-1) plt.plot(datasetLogDiffShifting) plt.plot(results_ARIMA.fittedvalues, color="red") print('RSS: %.4f'% sum((results_MA.fittedvalues- datasetLogDiffShifting["like"])**2)) predictions_ARIMA_diff = pd.Series(results_ARIMA.fittedvalues, copy=True) print(predictions_ARIMA_diff.head()) #Convert to cumulative sum predictions_ARIMA_diff_cumsum = predictions_ARIMA_diff.cumsum() print(predictions_ARIMA_diff_cumsum.head()) predictions_ARIMA_log = pd.Series(indexedDataset_logScale["like"].iloc[0], index=indexedDataset_logScale.index) predictions_ARIMA_log = predictions_ARIMA_log.add(predictions_ARIMA_diff_cumsum,fill_value=0) predictions_ARIMA_log.head() predictions_ARIMA = np.exp(predictions_ARIMA_log) plt.plot(indexedDataset) plt.plot(predictions_ARIMA) indexedDataset_logScale results_ARIMA.plot_predict(1,264) # x=results_ARIMA.forecast(steps=120)
-
Jeril about 5 yearscan you share the entire traceback / error
-
Admin about 5 yearsupdated the question bro! its there now
-
Jeril about 5 yearswhat do you get from the following code:
indexedDataset.isnull().sum()
-
Admin about 5 yearsah! its just a line to see how many null values are there in the data file
-
Jeril about 5 yearswhat do you understand from the error
-
Admin about 5 yearsIm new to python. Basically what I get is that That some data might be missing
-
Jeril about 5 yearswhat is the output that you get when you print this
indexedDataset.isnull().sum()
-
Admin about 5 yearsOMG! I cannot find the line
indexedDataset.isnull().sum()
in my code !!! -
Jeril about 5 yearsyou need to add it after this line
indexedDataset = dataset.set_index(['Date'])
-
Admin about 5 yearsI get the result as
like 10
I added the following line to the code and now the error is gone! But a new one is thereindexedDataset = indexedDataset[indexedDataset != 0]
The error now there isMissingDataError: exog contains inf or nans
-
Admin about 5 yearsMissingDataError Traceback (most recent call last) <ipython-input-5-35993c1e078a> in <module> 37 from statsmodels.tsa.stattools import adfuller 38 print("Results of Dickey-Fuller Test:") ---> 39 dftest = adfuller(indexedDataset["like"], autolag='AIC') 40 41 dfoutput = pd.Series(dftest[0:4],index=['Test Statistics','p-value', '#Lags Used','#Number of observations used'])
-
-
Admin about 5 yearsNot working ! I added the following line to the code and now the error is gone!
indexedDataset = indexedDataset[indexedDataset != 0]
-
Admin about 5 yearsBut now there is a new error !
MissingDataError Traceback (most recent call last) <ipython-input-7-35993c1e078a> in <module> 37 from statsmodels.tsa.stattools import adfuller 38 print("Results of Dickey-Fuller Test:") ---> 39 dftest = adfuller(indexedDataset["like"], autolag='AIC') 40 41 dfoutput = pd.Series(dftest[0:4],index=['Test Statistics','p-value', '#Lags Used','#Number of observations used'])
-
Jeril about 5 yearscan you share the entire traceback
-
Jeril about 5 yearsdid you try my solution
-
Jeril about 5 yearswhat do you get when you print this
indexedDataset.isnull().sum()
after the lineindexedDataset = indexedDataset.fillna(method='ffill')