Detect touching/overlapping circles/ellipses with OpenCV and Python
Solution 1
Here's my attempt at detecting the circles. In summary
- perform a BGR->HSV conversion and use the V channel for processing
V channel:
- threshold, apply morphological closing, then take the distance transform (I'll call it dist)
dist image:
- create a template. From the sizes of the circles in the image, a ~75 pixel radius disk looks reasonable. Take its distance transform and use it as the template (I'll call it temp)
temp image:
- perform template matching: dist * temp
dist * temp image:
- find the local maxima of the resulting image. Location of the maxima correspond to circle centers and max values correspond to their radii
Thresholding template matched image:
Detecting circles as local maxima:
I did this in C++ as I'm most comfortable with it. I think you can easily convert this to python if you find this useful. Note that the above images are not to scale. Hope this helps.
EDIT: Added the Python version
C++:
double min, max;
Point maxLoc;
Mat im = imread("04Bxy.jpg");
Mat hsv;
Mat channels[3];
// bgr -> hsv
cvtColor(im, hsv, CV_BGR2HSV);
split(hsv, channels);
// use v channel for processing
Mat& ch = channels[2];
// apply Otsu thresholding
Mat bw;
threshold(ch, bw, 0, 255, CV_THRESH_BINARY | CV_THRESH_OTSU);
// close small gaps
Mat kernel = getStructuringElement(MORPH_ELLIPSE, Size(3, 3));
Mat morph;
morphologyEx(bw, morph, CV_MOP_CLOSE, kernel);
// take distance transform
Mat dist;
distanceTransform(morph, dist, CV_DIST_L2, CV_DIST_MASK_PRECISE);
// add a black border to distance transformed image. we are going to do
// template matching. to get a good match for circles in the margin, we are adding a border
int borderSize = 75;
Mat distborder(dist.rows + 2*borderSize, dist.cols + 2*borderSize, dist.depth());
copyMakeBorder(dist, distborder,
borderSize, borderSize, borderSize, borderSize,
BORDER_CONSTANT | BORDER_ISOLATED, Scalar(0, 0, 0));
// create a template. from the sizes of the circles in the image,
// a ~75 radius disk looks reasonable, so the borderSize was selected as 75
Mat distTempl;
Mat kernel2 = getStructuringElement(MORPH_ELLIPSE, Size(2*borderSize+1, 2*borderSize+1));
// erode the ~75 radius disk a bit
erode(kernel2, kernel2, kernel, Point(-1, -1), 10);
// take its distance transform. this is the template
distanceTransform(kernel2, distTempl, CV_DIST_L2, CV_DIST_MASK_PRECISE);
// match template
Mat nxcor;
matchTemplate(distborder, distTempl, nxcor, CV_TM_CCOEFF_NORMED);
minMaxLoc(nxcor, &min, &max);
// threshold the resulting image. we should be able to get peak regions.
// we'll locate the peak of each of these peak regions
Mat peaks, peaks8u;
threshold(nxcor, peaks, max*.5, 255, CV_THRESH_BINARY);
convertScaleAbs(peaks, peaks8u);
// find connected components. we'll use each component as a mask for distance transformed image,
// then extract the peak location and its strength. strength corresponds to the radius of the circle
vector<vector<Point>> contours;
vector<Vec4i> hierarchy;
findContours(peaks8u, contours, hierarchy, CV_RETR_CCOMP, CV_CHAIN_APPROX_SIMPLE, Point(0, 0));
for(int idx = 0; idx >= 0; idx = hierarchy[idx][0])
{
// prepare the mask
peaks8u.setTo(Scalar(0, 0, 0));
drawContours(peaks8u, contours, idx, Scalar(255, 255, 255), -1);
// find the max value and its location in distance transformed image using mask
minMaxLoc(dist, NULL, &max, NULL, &maxLoc, peaks8u);
// draw the circles
circle(im, maxLoc, (int)max, Scalar(0, 0, 255), 2);
}
Python:
import cv2
im = cv2.imread('04Bxy.jpg')
hsv = cv2.cvtColor(im, cv2.COLOR_BGR2HSV)
th, bw = cv2.threshold(hsv[:, :, 2], 0, 255, cv2.THRESH_BINARY | cv2.THRESH_OTSU)
kernel = cv2.getStructuringElement(cv2.MORPH_ELLIPSE, (3, 3))
morph = cv2.morphologyEx(bw, cv2.MORPH_CLOSE, kernel)
dist = cv2.distanceTransform(morph, cv2.cv.CV_DIST_L2, cv2.cv.CV_DIST_MASK_PRECISE)
borderSize = 75
distborder = cv2.copyMakeBorder(dist, borderSize, borderSize, borderSize, borderSize,
cv2.BORDER_CONSTANT | cv2.BORDER_ISOLATED, 0)
gap = 10
kernel2 = cv2.getStructuringElement(cv2.MORPH_ELLIPSE, (2*(borderSize-gap)+1, 2*(borderSize-gap)+1))
kernel2 = cv2.copyMakeBorder(kernel2, gap, gap, gap, gap,
cv2.BORDER_CONSTANT | cv2.BORDER_ISOLATED, 0)
distTempl = cv2.distanceTransform(kernel2, cv2.cv.CV_DIST_L2, cv2.cv.CV_DIST_MASK_PRECISE)
nxcor = cv2.matchTemplate(distborder, distTempl, cv2.TM_CCOEFF_NORMED)
mn, mx, _, _ = cv2.minMaxLoc(nxcor)
th, peaks = cv2.threshold(nxcor, mx*0.5, 255, cv2.THRESH_BINARY)
peaks8u = cv2.convertScaleAbs(peaks)
contours, hierarchy = cv2.findContours(peaks8u, cv2.RETR_CCOMP, cv2.CHAIN_APPROX_SIMPLE)
peaks8u = cv2.convertScaleAbs(peaks) # to use as mask
for i in range(len(contours)):
x, y, w, h = cv2.boundingRect(contours[i])
_, mx, _, mxloc = cv2.minMaxLoc(dist[y:y+h, x:x+w], peaks8u[y:y+h, x:x+w])
cv2.circle(im, (int(mxloc[0]+x), int(mxloc[1]+y)), int(mx), (255, 0, 0), 2)
cv2.rectangle(im, (x, y), (x+w, y+h), (0, 255, 255), 2)
cv2.drawContours(im, contours, i, (0, 0, 255), 2)
cv2.imshow('circles', im)
Solution 2
I got some errors with your code @dhanuskha. I guess is because I am using a different version of CV. This code works with CV 3.0 in case you need it.
import cv2
im = cv2.imread('input.png')
hsv = cv2.cvtColor(im, cv2.COLOR_BGR2HSV)
th, bw = cv2.threshold(hsv[:, :, 2], 0, 255, cv2.THRESH_BINARY | cv2.THRESH_OTSU)
kernel = cv2.getStructuringElement(cv2.MORPH_ELLIPSE, (3, 3))
morph = cv2.morphologyEx(bw, cv2.MORPH_CLOSE, kernel)
dist = cv2.distanceTransform(morph, cv2.DIST_L2, cv2.DIST_MASK_PRECISE)
borderSize = 75
distborder = cv2.copyMakeBorder(dist, borderSize, borderSize, borderSize, borderSize,
cv2.BORDER_CONSTANT | cv2.BORDER_ISOLATED, 0)
gap = 10
kernel2 = cv2.getStructuringElement(cv2.MORPH_ELLIPSE, (2*(borderSize-gap)+1, 2*(borderSize-gap)+1))
kernel2 = cv2.copyMakeBorder(kernel2, gap, gap, gap, gap,
cv2.BORDER_CONSTANT | cv2.BORDER_ISOLATED, 0)
distTempl = cv2.distanceTransform(kernel2, cv2.DIST_L2, cv2.DIST_MASK_PRECISE)
nxcor = cv2.matchTemplate(distborder, distTempl, cv2.TM_CCOEFF_NORMED)
mn, mx, _, _ = cv2.minMaxLoc(nxcor)
th, peaks = cv2.threshold(nxcor, mx*0.5, 255, cv2.THRESH_BINARY)
peaks8u = cv2.convertScaleAbs(peaks)
_, contours, hierarchy = cv2.findContours(peaks8u, cv2.RETR_CCOMP, cv2.CHAIN_APPROX_SIMPLE)
peaks8u = cv2.convertScaleAbs(peaks) # to use as mask
for i in range(len(contours)):
x, y, w, h = cv2.boundingRect(contours[i])
_, mx, _, mxloc = cv2.minMaxLoc(dist[y:y+h, x:x+w], peaks8u[y:y+h, x:x+w])
cv2.circle(im, (int(mxloc[0]+x), int(mxloc[1]+y)), int(mx), (255, 0, 0), 2)
cv2.rectangle(im, (x, y), (x+w, y+h), (0, 255, 255), 2)
cv2.drawContours(im, contours, i, (0, 0, 255), 2)
cv2.imshow('circles', im)
cv2.waitKey(0)
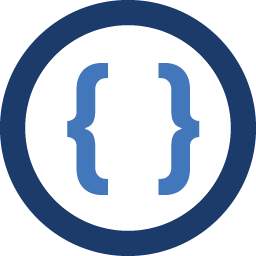
Admin
Updated on June 15, 2022Comments
-
Admin almost 2 years
i want to measure the circularity of circles (difference of the "circles" height and width or ellipse parameters). The circles are given in pictures as shown here:
After doing usual stuff like color2gray, thresholding and border detection, I get the following picture as shown:
With this, I already tried a lot of different things:
- List item Watershed with findContour (similar to this question) -> openCV detects the space between the circles as a closed contour and not the circles since they stick together not forming a closed contour
- same problem with fitEllipse. I get ellipses fitted on the black background contour and not in between.
- just trying to apply hough transforamtion (as in the code and the third picture shown) as well leads to strange results:
See the code here:
import sys import cv2 import numpy from scipy.ndimage import label # Application entry point #img = cv2.imread("02_adj_grey.jpg") img = cv2.imread("fuss02.jpg") # Pre-processing. img_gray = cv2.cvtColor(img, cv2.COLOR_BGR2GRAY) cv2.imwrite("SO_0_gray.png", img_gray) #_, img_bin = cv2.threshold(img_gray, 0, 255, cv2.THRESH_OTSU | cv2.THRESH_BINARY) _, img_bin = cv2.threshold(img_gray, 170, 255, cv2.THRESH_BINARY) cv2.imwrite("SO_1_threshold.png", img_bin) #blur = cv2.GaussianBlur(img,(5,5),0) img_bin = cv2.morphologyEx(img_bin, cv2.MORPH_CLOSE, numpy.ones((3, 3), dtype=int)) cv2.imwrite("SO_2_img_bin_morphoEx.png", img_bin) border = img_bin - cv2.erode(img_bin, None) cv2.imwrite("SO_3_border.png", border) circles = cv2.HoughCircles(border,cv2.cv.CV_HOUGH_GRADIENT,50,80, param1=80,param2=40,minRadius=10,maxRadius=150) print circles cimg = img for i in circles[0,:]: # draw the outer circle cv2.circle(cimg,(i[0],i[1]),i[2],(0,255,0),2) # draw the center of the circle cv2.circle(cimg,(i[0],i[1]),2,(0,0,255),3) cv2.putText(cimg,str(i[0])+str(',')+str(i[1]), (i[0],i[1]), cv2.FONT_HERSHEY_SIMPLEX, 0.4, 255) cv2.imwrite("SO_8_cimg.png", cimg)
Does anyone have an idea to improve my algorhitm or a complete different approach? I have been trying many different approaches but without luck so far. Thanks everyone for your help.