How do I get RSS from a linear model output
53,510
Here are some ways of computing the residual sum of squares (RSS) using the built-in anscombe
data set:
fm <- lm(y1 ~ x1+x2+x3, anscombe)
deviance(fm)
## [1] 13.76269
sum(resid(fm)^2)
## [1] 13.76269
anova(fm) # see the Residuals row of the Sum Sq column
## Analysis of Variance Table
##
## Response: y1
## Df Sum Sq Mean Sq F value Pr(>F)
## x1 1 27.510 27.5100 17.99 0.00217 **
## Residuals 9 13.763 1.5292
## ---
## Signif. codes: 0 ‘***’ 0.001 ‘**’ 0.01 ‘*’ 0.05 ‘.’ 0.1 ‘ ’ 1
anova(fm)["Residuals", "Sum Sq"]
## [1] 13.76269
with(summary(fm), df[2] * sigma^2)
## [1] 13.76269
Regarding the last one, note that summary(fm)$df[2]
and summary(fm)$sigma
are shown in the summary(fm)
output in case you want to calculate RSS using only a printout from summary
. In particular, for the output shown in the question df[2] = 116 and sigma = 1.928 so RSS = df[2] * sigma^2 = 116 * 1.928^2 = 431.1933 .
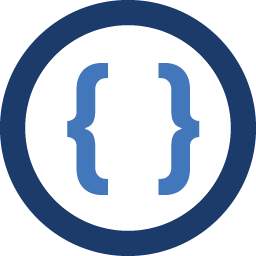
Author by
Admin
Updated on March 21, 2021Comments
-
Admin about 3 years
Below is a linear model output for a dataset consisting of a response variable and three explanatory variables. How do I get the RSS of the original regression?
Call: lm(formula = y ~ x1 + x2 + x3) Residuals: Min 1Q Median 3Q Max -4.9282 -1.3174 0.0059 1.3238 4.4560 Coefficients: Estimate Std. Error t value Pr(>|t|) (Intercept) -7.056057 1.963805 -3.593 0.000481 *** x1 3.058592 0.089442 34.196 < 2e-16 *** x2 -5.763410 0.168072 -34.291 < 2e-16 *** x3 0.000571 0.165153 0.003 0.997247 --- Signif. codes: 0 *** 0.001 ** 0.01 * 0.05 . 0.1 1 Residual standard error: 1.928 on 116 degrees of freedom Multiple R-squared: 0.9546,Adjusted R-squared: 0.9535 F-statistic: 814 on 3 and 116 DF, p-value: < 2.2e-16