how to implement early stopping in tensorflow
Solution 1
Here is my implementation of the early stopping u can adapt it:
The early stopping can be applied at certain stages of the training process, such as at the end of each epoch. Specifically; in my case; I monitor the test (validation) loss at each epoch and after the test loss has not improved after 20
epochs (self.require_improvement= 20
) , the training is interrupted.
You can set the max epochs to 10000 or 20000 or whatever you want (self.max_epochs = 10000
).
self.require_improvement= 20
self.max_epochs = 10000
Here is my training function where I use the early stopping:
def train(self):
# training data
train_input = self.Normalize(self.x_train)
train_output = self.y_train.copy()
#===============
save_sess=self.sess # this used to compare the result of previous sess with actual one
# ===============
#costs history :
costs = []
costs_inter=[]
# =================
#for early stopping :
best_cost=1000000
stop = False
last_improvement=0
# ================
n_samples = train_input.shape[0] # size of the training set
# ===============
#train the mini_batches model using the early stopping criteria
epoch = 0
while epoch < self.max_epochs and stop == False:
#train the model on the traning set by mini batches
#suffle then split the training set to mini-batches of size self.batch_size
seq =list(range(n_samples))
random.shuffle(seq)
mini_batches = [
seq[k:k+self.batch_size]
for k in range(0,n_samples, self.batch_size)
]
avg_cost = 0. # The average cost of mini_batches
step= 0
for sample in mini_batches:
batch_x = x_train.iloc[sample, :]
batch_y =train_output.iloc[sample, :]
batch_y = np.array(batch_y).flatten()
feed_dict={self.X: batch_x,self.Y:batch_y, self.is_train:True}
_, cost,acc=self.sess.run([self.train_step, self.loss_, self.accuracy_],feed_dict=feed_dict)
avg_cost += cost *len(sample)/n_samples
print('epoch[{}] step [{}] train -- loss : {}, accuracy : {}'.format(epoch,step, avg_cost, acc))
step += 100
#cost history since the last best cost
costs_inter.append(avg_cost)
#early stopping based on the validation set/ max_steps_without_decrease of the loss value : require_improvement
if avg_cost < best_cost:
save_sess= self.sess # save session
best_cost = avg_cost
costs +=costs_inter # costs history of the validatio set
last_improvement = 0
costs_inter= []
else:
last_improvement +=1
if last_improvement > self.require_improvement:
print("No improvement found during the ( self.require_improvement) last iterations, stopping optimization.")
# Break out from the loop.
stop = True
self.sess=save_sess # restore session with the best cost
## Run validation after every epoch :
print('---------------------------------------------------------')
self.y_validation = np.array(self.y_validation).flatten()
loss_valid, acc_valid = self.sess.run([self.loss_,self.accuracy_],
feed_dict={self.X: self.x_validation, self.Y: self.y_validation,self.is_train: True})
print("Epoch: {0}, validation loss: {1:.2f}, validation accuracy: {2:.01%}".format(epoch + 1, loss_valid, acc_valid))
print('---------------------------------------------------------')
epoch +=1
We can resume the important code here :
def train(self):
...
#costs history :
costs = []
costs_inter=[]
#for early stopping :
best_cost=1000000
stop = False
last_improvement=0
#train the mini_batches model using the early stopping criteria
epoch = 0
while epoch < self.max_epochs and stop == False:
...
for sample in mini_batches:
...
#cost history since the last best cost
costs_inter.append(avg_cost)
#early stopping based on the validation set/ max_steps_without_decrease of the loss value : require_improvement
if avg_cost < best_cost:
save_sess= self.sess # save session
best_cost = avg_cost
costs +=costs_inter # costs history of the validatio set
last_improvement = 0
costs_inter= []
else:
last_improvement +=1
if last_improvement > self.require_improvement:
print("No improvement found during the ( self.require_improvement) last iterations, stopping optimization.")
# Break out from the loop.
stop = True
self.sess=save_sess # restore session with the best cost
...
epoch +=1
Hope it will help someone :).
Solution 2
ValidationMonitor is marked as deprecated. it is not recommended. but you still can use it. here is a example of how to create one:
validation_monitor = monitors.ValidationMonitor(
input_fn=functools.partial(input_fn, subset="evaluation"),
eval_steps=128,
every_n_steps=88,
early_stopping_metric="accuracy",
early_stopping_rounds = 1000
)
and you can implement by yourself, here my my implementation:
if (loss_value < self.best_loss):
self.stopping_step = 0
self.best_loss = loss_value
else:
self.stopping_step += 1
if self.stopping_step >= FLAGS.early_stopping_step:
self.should_stop = True
print("Early stopping is trigger at step: {} loss:{}".format(global_step,loss_value))
run_context.request_stop()
Solution 3
Since TensorFlow version r1.10
early stopping hooks are available for the estimator API in early_stopping.py
(see github).
For example tf.contrib.estimator.stop_if_no_decrease_hook
(see docs)
Solution 4
For a custom training loop with tf.keras
, you can implement it like this:
def main(early_stopping, epochs=50):
loss_history = deque(maxlen=early_stopping + 1)
for epoch in range(epochs):
fit(epoch)
loss_history.append(test_loss.result().numpy())
if len(loss_history) > early_stopping:
if loss_history.popleft() < min(loss_history):
print(f'\nEarly stopping. No validation loss '
f'improvement in {early_stopping} epochs.')
break
At every epoch end, the validation loss is reconrded in a collections.deque
. Let's assume that early_stopping
is set to 3. Every epoch, the 4th last loss is compared to the last three losses. If there is no improvement in these 3 losses, then the loop is interrupted.
Here is the full code:
import os
os.environ['TF_CPP_MIN_LOG_LEVEL'] = '3'
import tensorflow_datasets as tfds
import tensorflow as tf
from collections import deque
data, info = tfds.load('iris', split='train',
as_supervised=True,
shuffle_files=True,
with_info=True)
dataset = data.shuffle(info.splits['train'].num_examples)
train_dataset = dataset.take(120).batch(4)
test_dataset = dataset.skip(120).take(30).batch(4)
model = tf.keras.Sequential([
tf.keras.layers.Dense(8, activation='relu'),
tf.keras.layers.Dense(16, activation='relu'),
tf.keras.layers.Dense(32, activation='relu'),
tf.keras.layers.Dense(info.features['label'].num_classes, activation='softmax')
])
loss_object = tf.keras.losses.SparseCategoricalCrossentropy(from_logits=True)
train_loss = tf.keras.metrics.Mean()
test_loss = tf.keras.metrics.Mean()
train_acc = tf.keras.metrics.SparseCategoricalAccuracy()
test_acc = tf.keras.metrics.SparseCategoricalAccuracy()
opt = tf.keras.optimizers.Adam(learning_rate=1e-3)
@tf.function
def train_step(inputs, labels):
with tf.GradientTape() as tape:
logits = model(inputs)
loss = loss_object(labels, logits)
gradients = tape.gradient(loss, model.trainable_variables)
opt.apply_gradients(zip(gradients, model.trainable_variables))
train_loss(loss)
train_acc(labels, logits)
@tf.function
def test_step(inputs, labels):
logits = model(inputs)
loss = loss_object(labels, logits)
test_loss(loss)
test_acc(labels, logits)
def fit(epoch):
template = 'Epoch {:>2} Train Loss {:.4f} Test Loss {:.4f} ' \
'Train Acc {:.2%} Test Acc {:.2%}'
train_loss.reset_states()
test_loss.reset_states()
train_acc.reset_states()
test_acc.reset_states()
for X_train, y_train in train_dataset:
train_step(X_train, y_train)
for X_test, y_test in test_dataset:
test_step(X_test, y_test)
print(template.format(
epoch + 1,
train_loss.result(),
test_loss.result(),
train_acc.result(),
test_acc.result()
))
def main(early_stopping, epochs=50):
loss_history = deque(maxlen=early_stopping + 1)
for epoch in range(epochs):
fit(epoch)
loss_history.append(test_loss.result().numpy())
if len(loss_history) > early_stopping:
if loss_history.popleft() < min(loss_history):
print(f'\nEarly stopping. No validation loss '
f'improvement in {early_stopping} epochs.')
break
if __name__ == '__main__':
main(epochs=100, early_stopping=3)
Here is the output:
Epoch 1 Train Loss 1.0368 Test Loss 0.9507 Train Acc 66.67% Test Acc 76.67%
Epoch 2 Train Loss 1.0013 Test Loss 0.9673 Train Acc 65.83% Test Acc 70.00%
Epoch 3 Train Loss 0.9582 Test Loss 1.0055 Train Acc 64.17% Test Acc 56.67%
Epoch 4 Train Loss 0.9116 Test Loss 0.8510 Train Acc 63.33% Test Acc 70.00%
Epoch 5 Train Loss 0.8401 Test Loss 0.8632 Train Acc 67.50% Test Acc 76.67%
Epoch 6 Train Loss 0.8114 Test Loss 0.7535 Train Acc 72.50% Test Acc 80.00%
Epoch 7 Train Loss 0.8105 Test Loss 0.8240 Train Acc 68.33% Test Acc 80.00%
Epoch 8 Train Loss 0.7956 Test Loss 0.7855 Train Acc 81.67% Test Acc 93.33%
Epoch 9 Train Loss 0.7740 Test Loss 0.8094 Train Acc 89.17% Test Acc 73.33%
Early stopping. No validation loss improvement in 3 epochs.
As you can see, the last best validation loss is at epoch 6, and then there are three losses after that, with no improvement. Then loop was therefore interrupted.
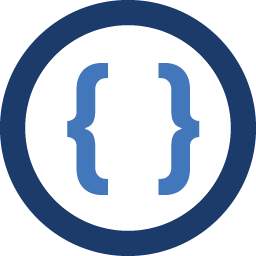
Admin
Updated on July 09, 2022Comments
-
Admin almost 2 years
def train(): # Model model = Model() # Loss, Optimizer global_step = tf.Variable(1, dtype=tf.int32, trainable=False, name='global_step') loss_fn = model.loss() optimizer = tf.train.AdamOptimizer(learning_rate=TrainConfig.LR).minimize(loss_fn, global_step=global_step) # Summaries summary_op = summaries(model, loss_fn) with tf.Session(config=TrainConfig.session_conf) as sess: # Initialized, Load state sess.run(tf.global_variables_initializer()) model.load_state(sess, TrainConfig.CKPT_PATH) writer = tf.summary.FileWriter(TrainConfig.GRAPH_PATH, sess.graph) # Input source data = Data(TrainConfig.DATA_PATH) loss = Diff() for step in xrange(global_step.eval(), TrainConfig.FINAL_STEP): mixed_wav, src1_wav, src2_wav, _ = data.next_wavs(TrainConfig.SECONDS, TrainConfig.NUM_WAVFILE, step) mixed_spec = to_spectrogram(mixed_wav) mixed_mag = get_magnitude(mixed_spec) src1_spec, src2_spec = to_spectrogram(src1_wav), to_spectrogram(src2_wav) src1_mag, src2_mag = get_magnitude(src1_spec), get_magnitude(src2_spec) src1_batch, _ = model.spec_to_batch(src1_mag) src2_batch, _ = model.spec_to_batch(src2_mag) mixed_batch, _ = model.spec_to_batch(mixed_mag) # Initializae our callback. #early_stopping_cb = EarlyStoppingCallback(val_acc_thresh=0.5) l, _, summary = sess.run([loss_fn, optimizer, summary_op], feed_dict={model.x_mixed: mixed_batch, model.y_src1: src1_batch, model.y_src2: src2_batch}) loss.update(l) print('step-{}\td_loss={:2.2f}\tloss={}'.format(step, loss.diff * 100, loss.value)) writer.add_summary(summary, global_step=step) # Save state if step % TrainConfig.CKPT_STEP == 0: tf.train.Saver().save(sess, TrainConfig.CKPT_PATH + '/checkpoint', global_step=step) writer.close()
I have this neural network code that separates music from a voice in a .wav file. how can I introduce an early stopping algorithm to stop the train section? I see some project that talks about a ValidationMonitor. Can someone help me?