Lisp and Prolog for Artificial Intelligence?
Solution 1
Can't really speak to Prolog, but here's why Lisp:
Lisp is a homoiconic language, which means that the code is expressed in the same form (s-expressions) as data structures in the language. i.e. "code is data". This has big advantages if you are writing code that modifies/manipulates other code, e.g. genetic algorithms or symbolic manipulation.
Lisp's macro system makes it well suited for defining problem-specific DSLs. Most Lisp developers effectively "extend the language" to do what they need. Again the fact that Lisp is homoiconic helps enormously here.
There is some historical connection, in that Lisp became popular at about the same time as a lot of the early AI research. Some interesting facts in this thread.
Lisp works pretty well as a functional programming language. This is quite a good domain fit for AI (where you are often just trying to get the machine to learn how to produce the correct output for a given input).
Subjective view: Lisp seems to appeal to people with a mathematical mindset, which happens to be exactly whet you need for a lot of modern AI..... this is possible due to the fact that Lisp is pretty closely related to the untyped lambda calculus
I'm doing some AI/machine learning work at the moment, and chose Clojure (a modern Lisp on the JVM) pretty much for the above reasons.
Solution 2
The question has already been answered for Lisp, so I'll just comment on Prolog.
Prolog was designed for two things: natural language processing and logical reasoning. In the GOFAI paradigm of the early 1970s, when Prolog was invented, this meant:
- constructing symbolic grammars for natural language that would be used to construct logical representations of sentences/utterances;
- using these representations and logical axioms (not necessarily those of classical logic) to infer new facts;
- using similar grammars to translate logical representation back into language.
Prolog is very good at this and is used in the ISS for exactly such a task. The approach got discredited though, because
- "all grammars leak": no grammar can catch all the rules and exceptions in a language;
- the more detailed the grammar, the higher the complexity (both big O and practical) of parsing;
- logical reasoning is both inadequate and unnecessary for many practical tasks;
- statistical approaches to NLP, i.e. "word counting", have proven much more robust. With the rise of the Internet, adequate datasets are available to get the statistics NLP developers need. At the same time, memory and disk costs has declined while processing power is still relatively expensive.
Only recently have NLP researchers developed somewhat practical combined symbolic-statistical approaches, sometimes using Prolog. The rest of the world uses Java, C++ or Python, for which you can more easily find libraries, tools and non-PhD programmers. The fact that I/O and arithmetic are unwieldy in Prolog doesn't help its acceptance.
Prolog is now mostly confined to domain-specific applications involving NLP and constraint reasoning, where it does seem to fare quite well. Still, few software companies will advertise with "built on Prolog technology" since the language got a bad name for not living up to the promise of "making AI easy."
(I'd like to add that I'm a great fan of Prolog, but even I only use it for prototyping.)
Solution 3
Lisp had an advantage when we believed AI was symbol manipulation and things like Ontologies. Prolog had an advantage when we believed AI as logic, and Unification was the tricky operation. But neither of these provide any advantage for any of the current contenders for "AI": Statistical AI is about sparse arrays. Neural networks of all kinds, including deep learning, is about oceans of nodes connected with links. Model Free Methods (many kinds of machine learning, evolutionary methods, etc) are also very simple. The complexity is emergent, so you don't have to worry about it. Write a simple base that can learn what it needs to learn. In either of these cases, any general purpose language will do. Arguments can even be made that most Neural Network approaches are so simple that C++ would be overkill.
Use the language that allows you to most easily hire the best programmers for the task.
Solution 4
It maybe a good idea to recall the motivations for Prolog: Logic for problem solving and to understand reasoning, human or machine like. This is an ongoing project and even though Prolog is one of its finest result, is not its final. We keep looking for better languages to represent knowledge. Check the latest book by Bob Kowalski: how to be artificially intelligent.
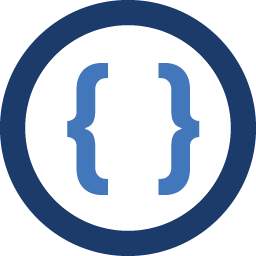
Admin
Updated on March 06, 2020Comments
-
Admin over 4 years
Now since i've taken a class 3 years ago in A.I. im clearly proficient enough to ask this question......just kidding just kidding ;)
but seriously, what is it about these languages that make them so popular for A.I. research. Even though A.I. research is "old"...it's came probably the longest way in the past 5-10 years it seems like.... Is it because the languages were somewhat "designed" around the concept of A.I. , or just that we have nothing really better to use right now?
I ask this because I've always found it quite interesting, and Im just kinda curious. If im entirely wrong and they use different languages I would love to know what all they use. I mean i can understand prolog, especially with Sentient/Propositional Logic and Fuzzy logic. but I dont understand "Why" we would use Lisp...and even what else A.I. researchers would use to do machine learning etc.
Any articles/books on the subject matter is helpful too :)
-
Admin over 13 yearsI've only had one experience in college with Lisp, and it was mostly (((((((((((((((((((this)))))))))))))))))))))))))))))))))))))). Kinda off putting. :(
-
mikera over 13 yearsah yeah, the dreaded brackets. this put me off for a while too.... but once I figured out why they were there (basically - they are a very elegant, consistent and minimal syntax for function application in keeping with the homoiconic design of the language) it all made sense and I was kicking myself for not learning Lisp earlier.
-
Ken over 13 yearsSauron: Too many close-parens? Seriously, write a function in Lisp, and then write the same function in another language, and count the grouping characters. I found that for Java, if you add all the
[]{}()
together, you end up with several times more than the parens in Lisp -- so this classic complaint is really "it's too concise and consistent". I think professors of Lisp classes should make everyone do one exercise in both Lisp and Java/C/whatever just to demonstrate that it's that way for a reason. (Have you ever tried doing SICP in Java? Yow.) -
ypercubeᵀᴹ over 13 years@Ken: you are probably right about
[]{}()
in Java. That's one reason Python appeals to many :) -
2rs2ts about 13 yearsAdopting a standard formatting style for your code, regardless of the language, will help your code to be more readable. Parens in LISP can be treated like squiggly brackets in C/Java and once you start indenting your expressions using them, you'll find that LISP is just as readable!
-
2rs2ts about 13 yearsThank you for your answer - I'm currently researching Prolog against LISP for AI programming for my class presentation and this helps a lot! I haven't even seen this "all grammars leak" concept yet in my research.
-
Fred Foo about 13 years@user691859: I find that highly surprising, given that the quote actually has a much broader scope than just grammar. Much in cognitive science is hard to express in rules and consequently rule-based approaches in AI have largely yielded to statistics and machine learning.
-
2rs2ts about 13 yearsThe concept was explained to me in CS Theory and Programming Language Concepts to explain why there is no CNF/BNF definition for English, but I've never heard that exact phrase nor have I seen that in my research. I actually haven't read yet that rule-based approaches have yielded to statistics and machine learning! My presentation is going to be weakly based, heh.
-
Admin almost 11 years"code that modifies/manipulates other code, e.g. genetic algorithms or symbolic manipulation" Really?
-
mikera almost 11 years@arbautjc - yes, really :-) . As an example, here's a library that a student implemented (as a summer GSoC project) that adds symbolic mathematical computation to Clojure - github.com/clojure-numerics/expresso - including symbolic differentiation, matrix maths, equation solving, expression simplification etc.
-
Admin almost 11 yearsThank you, I'll have a look at this. I was actually surprised by your statement that genetic algorithms or symbolic manipulation modify code, but I guess it depends on the implementation. I can imagine implementation than only manipulate data. Your last point (and most of the others) apply to OCaml too, and this reminds me of why Yaron Minsky chose this language at Jane Street Capital. But I confess I prefer Lisp :-)
-
Fattie over 9 yearsAn outstanding answer.
-
kisai about 5 yearsI actually like the parenthesis, i doesn't put me off because you will never find something like (((((((((((((((((((this)))))))))))))))))))))))))))))))))))))) to me, having the regular syntax of lisp makes it so easy and reduces the cognitive load of multiple syntax special cases. Nesting of parenthesis is handled by any code editor pretty well, like Atom or vscode, obviously emacs is better but you don't need to.
-
kisai about 5 yearsThe real connection comes from MIT's AI lab, where John McCarthy was one of the founders was AI researcher and created the term Artificial Intelligence, and with other he was on the AI school of Symbolic AI, Lisp was designed for this, Homoiconicity is part of Symbolic Programming which is what McCarthy used for Symbolic AI based on psychology.