Reusing model fitted by cross_val_score in sklearn using joblib
Solution 1
The real reason your model is not fitted is that the function cross_val_score
first copies your model before fitting the copy : Source link
So your original model has not been fitted.
Solution 2
It's not quite correct that cross-validation has to fit your model; rather a k-fold cross validation fits your model k times on partial data sets. If you want the model itself, you actually need to fit the model again on the whole dataset; this actually isn't part of the cross-validation process. So it actually wouldn't be redundant to call
alg.fit(data, labels)
to fit your model after your cross validation.
Another approcach would be rather than using the specialized function cross_val_score
, you could think of this as a special case of a cross-validated grid search (with a single point in the parameter space). In this case GridSearchCV
will by default refit the model over the entire dataset (it has a parameter refit=True
), and also has predict
and predict_proba
methods in its API.
Related videos on Youtube
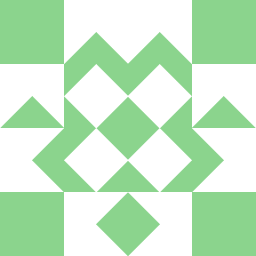
user
Updated on April 11, 2020Comments
-
user over 3 years
I created the following function in python:
def cross_validate(algorithms, data, labels, cv=4, n_jobs=-1): print "Cross validation using: " for alg, predictors in algorithms: print alg print # Compute the accuracy score for all the cross validation folds. scores = cross_val_score(alg, data, labels, cv=cv, n_jobs=n_jobs) # Take the mean of the scores (because we have one for each fold) print scores print("Cross validation mean score = " + str(scores.mean())) name = re.split('\(', str(alg)) filename = str('%0.5f' %scores.mean()) + "_" + name[0] + ".pkl" # We might use this another time joblib.dump(alg, filename, compress=1, cache_size=1e9) filenameL.append(filename) try: move(filename, "pkl") except: os.remove(filename) print return
I thought that in order to do cross validation, sklearn had to fit your function.
However, when I try to use it later (f is the pkl file I saved above in
joblib.dump(alg, filename, compress=1, cache_size=1e9))
:alg = joblib.load(f) predictions = alg.predict_proba(train_data[predictors]).astype(float)
I get no error in the first line (so it looks like the load is working), but then it tells me
NotFittedError: Estimator not fitted, call
fitbefore exploiting the model.
on the following line.What am I doing wrong? Can't I reuse the model fitted to calculate the cross-validation? I looked at Keep the fitted parameters when using a cross_val_score in scikits learn but either I don't understand the answer, or it is not what I am looking for. What I want is to save the whole model with joblib so that I can the use it later without re-fitting.
-
Jacquot over 5 yearsthat is just not true. Of course cross-validation has to fit your model, whether it is on partial data sets or on the whole, doesn't make a difference regarding the 'fitted' character of the model