selecting across multiple columns with python pandas?
Solution 1
I encourage you to pose these questions on the mailing list, but in any case, it's still a very much low level affair working with the underlying NumPy arrays. For example, to select rows where the value in any column exceed, say, 1.5 in this example:
In [11]: df
Out[11]:
A B C D
2000-01-03 -0.59885 -0.18141 -0.68828 -0.77572
2000-01-04 0.83935 0.15993 0.95911 -1.12959
2000-01-05 2.80215 -0.10858 -1.62114 -0.20170
2000-01-06 0.71670 -0.26707 1.36029 1.74254
2000-01-07 -0.45749 0.22750 0.46291 -0.58431
2000-01-10 -0.78702 0.44006 -0.36881 -0.13884
2000-01-11 0.79577 -0.09198 0.14119 0.02668
2000-01-12 -0.32297 0.62332 1.93595 0.78024
2000-01-13 1.74683 -1.57738 -0.02134 0.11596
2000-01-14 -0.55613 0.92145 -0.22832 1.56631
2000-01-17 -0.55233 -0.28859 -1.18190 -0.80723
2000-01-18 0.73274 0.24387 0.88146 -0.94490
2000-01-19 0.56644 -0.49321 1.17584 -0.17585
2000-01-20 1.56441 0.62331 -0.26904 0.11952
2000-01-21 0.61834 0.17463 -1.62439 0.99103
2000-01-24 0.86378 -0.68111 -0.15788 -0.16670
2000-01-25 -1.12230 -0.16128 1.20401 1.08945
2000-01-26 -0.63115 0.76077 -0.92795 -2.17118
2000-01-27 1.37620 -1.10618 -0.37411 0.73780
2000-01-28 -1.40276 1.98372 1.47096 -1.38043
2000-01-31 0.54769 0.44100 -0.52775 0.84497
2000-02-01 0.12443 0.32880 -0.71361 1.31778
2000-02-02 -0.28986 -0.63931 0.88333 -2.58943
2000-02-03 0.54408 1.17928 -0.26795 -0.51681
2000-02-04 -0.07068 -1.29168 -0.59877 -1.45639
2000-02-07 -0.65483 -0.29584 -0.02722 0.31270
2000-02-08 -0.18529 -0.18701 -0.59132 -1.15239
2000-02-09 -2.28496 0.36352 1.11596 0.02293
2000-02-10 0.51054 0.97249 1.74501 0.20525
2000-02-11 0.10100 0.27722 0.65843 1.73591
In [12]: df[(df.values > 1.5).any(1)]
Out[12]:
A B C D
2000-01-05 2.8021 -0.1086 -1.62114 -0.2017
2000-01-06 0.7167 -0.2671 1.36029 1.7425
2000-01-12 -0.3230 0.6233 1.93595 0.7802
2000-01-13 1.7468 -1.5774 -0.02134 0.1160
2000-01-14 -0.5561 0.9215 -0.22832 1.5663
2000-01-20 1.5644 0.6233 -0.26904 0.1195
2000-01-28 -1.4028 1.9837 1.47096 -1.3804
2000-02-10 0.5105 0.9725 1.74501 0.2052
2000-02-11 0.1010 0.2772 0.65843 1.7359
Multiple conditions have to be combined using &
or |
(and parentheses!):
In [13]: df[(df['A'] > 1) | (df['B'] < -1)]
Out[13]:
A B C D
2000-01-05 2.80215 -0.1086 -1.62114 -0.2017
2000-01-13 1.74683 -1.5774 -0.02134 0.1160
2000-01-20 1.56441 0.6233 -0.26904 0.1195
2000-01-27 1.37620 -1.1062 -0.37411 0.7378
2000-02-04 -0.07068 -1.2917 -0.59877 -1.4564
I'd be very interested to have some kind of query API to make these kinds of things easier
Solution 2
There are at least a few approaches to shortening the syntax for this in Pandas, until it gets a full query API down the road (perhaps I'll try to join the github project and do this is time permits and if no one else already has started).
One method to shorten the syntax a little is given below:
inds = df.apply(lambda x: x["A"]>10 and x["B"]<5, axis=1)
print df[inds].to_string()
To fully solve this, one would need to build something like the SQL select and where clauses into Pandas. This is not trivial at all, but one stab that I think might work for this is to use the Python operator
built-in module. This allows you to treat things like greater-than as functions instead of symbols. So you could do the following:
def pandas_select(dataframe, select_dict):
inds = dataframe.apply(lambda x: reduce(lambda v1,v2: v1 and v2,
[elem[0](x[key], elem[1])
for key,elem in select_dict.iteritems()]), axis=1)
return dataframe[inds]
Then a test example like yours would be to do the following:
import operator
select_dict = {
"A":(operator.gt,10),
"B":(operator.lt,5)
}
print pandas_select(df, select_dict).to_string()
You can shorten the syntax even further by either building in more arguments to pandas_select
to handle the different common logical operators automatically, or by importing them into the namespace with shorter names.
Note that the pandas_select
function above only works with logical-and chains of constraints. You'd have to modify it to get different logical behavior. Or use not
and DeMorgan's Laws.
Solution 3
A query feature has been added to Pandas since this question was asked and answered. An example is given below.
Given this sample data frame:
periods = 8
dates = pd.date_range('20170101', periods=periods)
rand_df = pd.DataFrame(np.random.randn(periods,4), index=dates,
columns=list('ABCD'))
The query syntax as follows will allow you to use multiple filters, like a "WHERE" clause in a select statement.
rand_df.query("A < 0 or B < 0")
See the Pandas documentation for additional details.
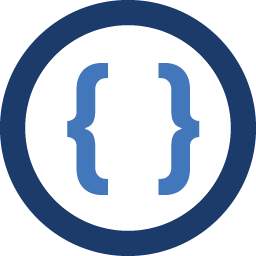
Admin
Updated on October 21, 2020Comments
-
Admin over 3 years
I have a dataframe
df
in pandas that was built usingpandas.read_table
from a csv file. The dataframe has several columns and it is indexed by one of the columns (which is unique, in that each row has a unique value for that column used for indexing.)How can I select rows of my dataframe based on a "complex" filter applied to multiple columns? I can easily select out the slice of the dataframe where column
colA
is greater than 10 for example:df_greater_than10 = df[df["colA"] > 10]
But what if I wanted a filter like: select the slice of
df
where any of the columns are greater than 10?Or where the value for
colA
is greater than 10 but the value forcolB
is less than 5?How are these implemented in pandas? Thanks.
-
Admin over 12 yearsThanks again.Will post future questions on mailing list. But for now, what if you wanted to do this programmatically? You had a list of column labels... how could you get that into the '|' notation? E.g. if
labels = ['A', 'B', 'C', ...']
-
Admin over 12 yearsTo clarify: The
any(1)
approach wouldn't work if you had other values in the table that you didn't want to filter. Suppose there are many columns and you only want theany
to apply to a subset of them (you know the subset's labels). -
David Braun almost 11 yearsIf I have a list ['Alice', 'Bob', 'Carl'] how can I generate the dictionary to select items where dataframe['A'] is in my list?
-
ely almost 11 yearsIf the list is
a = ['Alice', 'Bob', 'Carl']
and the overall data frame is calleddf
, then you can do this:df[df.A.isin(a)]
and it will sub-select the row indices where the set membership condition is true for elements of columnA
. Expanding the mini domain-specific language I made above for expressing logicals to have this option with simple syntax will probably be an uncomfortable chore. -
RuiDC over 10 yearsperhaps see also the forthcoming (pandas 0.13) query method: pandas.pydata.org/pandas-docs/dev/… and also stackoverflow.com/questions/18521037/…
-
Freek Wiekmeijer about 5 years
df.apply(lambda row: ..., axis=1)
is flexible but slow. -
ely about 5 years@FreekWiekmeijer That's true. In my experience, many people try to optimize away use of
lambda
or explicit iteration in pandas extremely prematurely, trying to refactor code into brittle and indecipherable vectorized operations right off the bat. Most use cases don't benefit much from this, and frankly you're better off writing the code in the "dumb" "obvious" way and splitting the data frame to use multiprocessing to speed something up, etc., than to commit too early to pandas legalese.