OpenCV / Python : multi-threading for live facial recognition
Solution 1
Performance issue was due to a bad compilation of dlib. Do not use pip install dlib
which runs very very slowly for some reason compared to the proper compilation. I went from almost 10 seconds lag to about 2 seconds this way. So finally I didn't need multi-threading/processing, but I'm working on it to enhance the speed even more. Thanks for the help :)
Solution 2
i tried a simplified approach like P.Ro mentioned in his answer with processes writing to an output queue but somehow the queue got locked most of the time because all the processes wrote to it at the same time. (just my guess) i probably did something wrong.
in the end i ended up using pipes.
the code is nasty. but if i was me a few hours ago. i would still be glad to find an example that actually runs without effort.
from multiprocessing import Process, Queue, Manager,Pipe
import multiprocessing
import face_recognition as fik
import cv2
import time
video_input = 0
obama_image = fik.load_image_file("obama.png")
obama_face_encoding = fik.face_encodings(obama_image)[0]
quality = 0.7
def f(id,fi,fl):
import face_recognition as fok
while True:
small_frame = fi.get()
print("running thread"+str(id))
face_locations = fok.face_locations(small_frame)
if(len(face_locations)>0):
print(face_locations)
for (top7, right7, bottom7, left7) in face_locations:
small_frame_c = small_frame[top7:bottom7, left7:right7]
fl.send(small_frame_c)
fps_var =0
if __name__ == '__main__':
multiprocessing.set_start_method('spawn')
# global megaman
with Manager() as manager:
video_capture = cv2.VideoCapture(video_input)
fi = Queue(maxsize=14)
threads = 8
proc = []
parent_p = []
thread_p = []
# procids = range(0,threads)
for t in range(0,threads):
p_t,c_t = Pipe()
parent_p.append(p_t)
thread_p.append(c_t)
print(t)
proc.append(Process(target=f, args=(t,fi,thread_p[t])))
proc[t].start()
useframe = False
frame_id = 0
while True:
# Grab a single frame of video
ret, frame = video_capture.read()
effheight, effwidth = frame.shape[:2]
if effwidth < 20:
break
# Resize frame of video to 1/4 size for faster face recognition processing
xxx = 930
yyy = 10/16 #0.4234375
small_frame = cv2.resize(frame, (xxx, int(xxx*yyy)))
if frame_id%2 == 0:
if not fi.full():
fi.put(small_frame)
print(frame_id)
cv2.imshow('Video', small_frame)
print("FPS: ", int(1.0 / (time.time() - fps_var)))
fps_var = time.time()
#GET ALL DETECTIONS
for t in range(0,threads):
if parent_p[t].poll():
small_frame_c = parent_p[t].recv()
cv2.imshow('recc', small_frame_c)
height34, width34 = small_frame_c.shape[:2]
# print fsizeee
if(width34<20):
print("face 2 small")
print(width34)
break
face_encodings_cam = fik.face_encodings(small_frame_c,[(0, width34, height34, 0)])
match = fik.compare_faces([obama_face_encoding], face_encodings_cam[0])
name = "Unknown"
if match[0]:
name = "Barack"
print(name)
break
frame_id += 1
# Hit 'q' on the keyboard to quit!
if cv2.waitKey(1) & 0xFF == ord('q'):
break
Solution 3
Do not have much experience with using ThreadPool, but I always just use Process like shown below. You should be able to easily edit this code to fit your needs. I wrote this with your implementation in mind.
This code will get the number of cores and start however many worker processes that will all be implementing the desired function in parallel. They all share a Queue of frames for input and all put to the same output Queue for the main to get and show. Each Queue has a maximum size, in this case 5. This ensures that despite the CPU time it takes to process, it will always be relatively live time.
import numpy as np
import cv2
from multiprocessing import Process, Queue
import time
#from common import clock, draw_str, StatValue
#import video
class Canny_Process(Process):
def __init__(self,frame_queue,output_queue):
Process.__init__(self)
self.frame_queue = frame_queue
self.output_queue = output_queue
self.stop = False
#Initialize your face detectors here
def get_frame(self):
if not self.frame_queue.empty():
return True, self.frame_queue.get()
else:
return False, None
def stopProcess(self):
self.stop = True
def canny_frame(self,frame):
# some intensive computation...
gray = cv2.cvtColor(frame, cv2.COLOR_BGR2GRAY)
edges = cv2.Canny(gray, 50, 100)
#To simulate CPU Time
#############################
for i in range(1000000):
x = 546*546
res = x/(i+1)
#############################
'REPLACE WITH FACE DETECT CODE HERE'
if self.output_queue.full():
self.output_queue.get_nowait()
self.output_queue.put(edges)
def run(self):
while not self.stop:
ret, frame = self.get_frame()
if ret:
self.canny_frame(frame)
if __name__ == '__main__':
frame_sum = 0
init_time = time.time()
def put_frame(frame):
if Input_Queue.full():
Input_Queue.get_nowait()
Input_Queue.put(frame)
def cap_read(cv2_cap):
ret, frame = cv2_cap.read()
if ret:
put_frame(frame)
cap = cv2.VideoCapture(0)
threadn = cv2.getNumberOfCPUs()
threaded_mode = True
process_list = []
Input_Queue = Queue(maxsize = 5)
Output_Queue = Queue(maxsize = 5)
for x in range((threadn -1)):
canny_process = Canny_Process(frame_queue = Input_Queue,output_queue = Output_Queue)
canny_process.daemon = True
canny_process.start()
process_list.append(canny_process)
ch = cv2.waitKey(1)
cv2.namedWindow('Threaded Video', cv2.WINDOW_NORMAL)
while True:
cap_read(cap)
if not Output_Queue.empty():
result = Output_Queue.get()
cv2.imshow('Threaded Video', result)
ch = cv2.waitKey(5)
if ch == ord(' '):
threaded_mode = not threaded_mode
if ch == 27:
break
cv2.destroyAllWindows()
This should do the trick just change my canny function to do your face detection. I wrote this from your code and compared the two. This is significantly faster. I am using multiprocessing.Process here. In python processes are truly parallel and threads are not quite because of the GIL. I am using 2 queues to send data back and forth between the main and the processes. Queues are both Thread and Process safe.
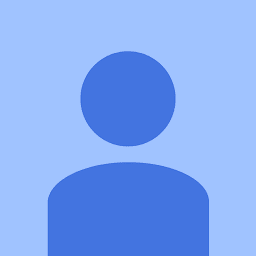
Simon
Updated on June 07, 2022Comments
-
Simon almost 2 years
I'm using OpenCv and Dlib to execute facial recognition w/ landmarks, live from the webcam stream. The language is Python. It works fine on my macbook laptop, but I need it to run from a desktop computer 24/7. The computer is a PC Intel® Core™2 Quad CPU Q6600 @ 2.40GHz 32bit running Debian Jessie. The drop in performance is drastic : there is a 10 seconds delay due to processing !
I therefore looked into multi-threading to gain performance :
- I first tried the sample code by OpenCv, and the result is great! All four cores hit 100%, and the performance is much better.
- I then replaced the frame processing code with my code, and it doesn't improve performance at all ! Only one core hits the 100%, the other ones stay very low. I even think it's worse with multi-threading on.
I got the facial landmark code from the dlib sample code. I know it can probably be optimized, but I want to understand why am I not able to use my (old) computer's full power with multi-threading ?
I'll drop my code below, thanks a lot for reading :)
from __future__ import print_function import numpy as np import cv2 import dlib from multiprocessing.pool import ThreadPool from collections import deque from common import clock, draw_str, StatValue import video class DummyTask: def __init__(self, data): self.data = data def ready(self): return True def get(self): return self.data if __name__ == '__main__': import sys print(__doc__) try: fn = sys.argv[1] except: fn = 0 cap = video.create_capture(fn) #Face detector detector = dlib.get_frontal_face_detector() #Landmarks shape predictor predictor = dlib.shape_predictor("landmarks/shape_predictor_68_face_landmarks.dat") # This is where the facial detection takes place def process_frame(frame, t0, detector, predictor): # some intensive computation... gray = cv2.cvtColor(frame, cv2.COLOR_BGR2GRAY) clahe = cv2.createCLAHE(clipLimit=2.0, tileGridSize=(8,8)) clahe_image = clahe.apply(gray) detections = detector(clahe_image, 1) for k,d in enumerate(detections): shape = predictor(clahe_image, d) for i in range(1,68): #There are 68 landmark points on each face cv2.circle(frame, (shape.part(i).x, shape.part(i).y), 1, (0,0,255), thickness=2) return frame, t0 threadn = cv2.getNumberOfCPUs() pool = ThreadPool(processes = threadn) pending = deque() threaded_mode = True latency = StatValue() frame_interval = StatValue() last_frame_time = clock() while True: while len(pending) > 0 and pending[0].ready(): res, t0 = pending.popleft().get() latency.update(clock() - t0) draw_str(res, (20, 20), "threaded : " + str(threaded_mode)) draw_str(res, (20, 40), "latency : %.1f ms" % (latency.value*1000)) draw_str(res, (20, 60), "frame interval : %.1f ms" % (frame_interval.value*1000)) cv2.imshow('threaded video', res) if len(pending) < threadn: ret, frame = cap.read() t = clock() frame_interval.update(t - last_frame_time) last_frame_time = t if threaded_mode: task = pool.apply_async(process_frame, (frame.copy(), t, detector, predictor)) else: task = DummyTask(process_frame(frame, t, detector, predictor)) pending.append(task) ch = cv2.waitKey(1) if ch == ord(' '): threaded_mode = not threaded_mode if ch == 27: break cv2.destroyAllWindows()