RuntimeError: expected scalar type Long but found Float
58,415
LongTensor
is synonymous with integer. PyTorch won't accept a FloatTensor
as categorical target, so it's telling you to cast your tensor to LongTensor
. This is how you should change your target dtype:
Yt_train = Yt_train.type(torch.LongTensor)
This is very well documented on the PyTorch website, you definitely won't regret spending a minute or two reading this page. PyTorch essentially defines nine CPU tensor types and nine GPU tensor types:
╔══════════════════════════╦═══════════════════════════════╦════════════════════╦═════════════════════════╗
║ Data type ║ dtype ║ CPU tensor ║ GPU tensor ║
╠══════════════════════════╬═══════════════════════════════╬════════════════════╬═════════════════════════╣
║ 32-bit floating point ║ torch.float32 or torch.float ║ torch.FloatTensor ║ torch.cuda.FloatTensor ║
║ 64-bit floating point ║ torch.float64 or torch.double ║ torch.DoubleTensor ║ torch.cuda.DoubleTensor ║
║ 16-bit floating point ║ torch.float16 or torch.half ║ torch.HalfTensor ║ torch.cuda.HalfTensor ║
║ 8-bit integer (unsigned) ║ torch.uint8 ║ torch.ByteTensor ║ torch.cuda.ByteTensor ║
║ 8-bit integer (signed) ║ torch.int8 ║ torch.CharTensor ║ torch.cuda.CharTensor ║
║ 16-bit integer (signed) ║ torch.int16 or torch.short ║ torch.ShortTensor ║ torch.cuda.ShortTensor ║
║ 32-bit integer (signed) ║ torch.int32 or torch.int ║ torch.IntTensor ║ torch.cuda.IntTensor ║
║ 64-bit integer (signed) ║ torch.int64 or torch.long ║ torch.LongTensor ║ torch.cuda.LongTensor ║
║ Boolean ║ torch.bool ║ torch.BoolTensor ║ torch.cuda.BoolTensor ║
╚══════════════════════════╩═══════════════════════════════╩════════════════════╩═════════════════════════╝
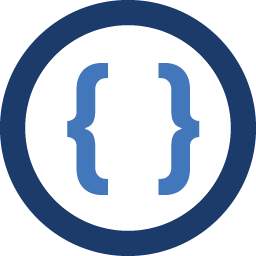
Author by
Admin
Updated on January 23, 2021Comments
-
Admin over 3 years
I can't get the dtypes to match, either the loss wants long or the model wants float if I change my tensors to long. The shape of the tensors are 42000, 1, 28, 28 and 42000. I'm not sure where I can change what dtypes are required for the model or loss.
I'm not sure if dataloader is required, using Variable didn't work either.
dataloaders_train = torch.utils.data.DataLoader(Xt_train, batch_size=64) dataloaders_test = torch.utils.data.DataLoader(Yt_train, batch_size=64) class Network(nn.Module): def __init__(self): super().__init__() self.hidden = nn.Linear(42000, 256) self.output = nn.Linear(256, 10) self.sigmoid = nn.Sigmoid() self.softmax = nn.Softmax(dim=1) def forward(self, x): x = self.hidden(x) x = self.sigmoid(x) x = self.output(x) x = self.softmax(x) return x model = Network() input_size = 784 hidden_sizes = [28, 64] output_size = 10 model = nn.Sequential(nn.Linear(input_size, hidden_sizes[0]), nn.ReLU(), nn.Linear(hidden_sizes[0], hidden_sizes[1]), nn.ReLU(), nn.Linear(hidden_sizes[1], output_size), nn.Softmax(dim=1)) print(model) criterion = nn.NLLLoss() optimizer = optim.SGD(model.parameters(), lr=0.003) epochs = 5 for e in range(epochs): running_loss = 0 for images, labels in zip(dataloaders_train, dataloaders_test): images = images.view(images.shape[0], -1) #images, labels = Variable(images), Variable(labels) print(images.dtype) print(labels.dtype) optimizer.zero_grad() output = model(images) loss = criterion(output, labels) loss.backward() optimizer.step() running_loss += loss.item() else: print(f"Training loss: {running_loss}")
Which gives
RuntimeError Traceback (most recent call last) <ipython-input-128-68109c274f8f> in <module> 11 12 output = model(images) ---> 13 loss = criterion(output, labels) 14 loss.backward() 15 optimizer.step() /opt/conda/lib/python3.6/site-packages/torch/nn/modules/module.py in __call__(self, *input, **kwargs) 530 result = self._slow_forward(*input, **kwargs) 531 else: --> 532 result = self.forward(*input, **kwargs) 533 for hook in self._forward_hooks.values(): 534 hook_result = hook(self, input, result) /opt/conda/lib/python3.6/site-packages/torch/nn/modules/loss.py in forward(self, input, target) 202 203 def forward(self, input, target): --> 204 return F.nll_loss(input, target, weight=self.weight, ignore_index=self.ignore_index, reduction=self.reduction) 205 206 /opt/conda/lib/python3.6/site-packages/torch/nn/functional.py in nll_loss(input, target, weight, size_average, ignore_index, reduce, reduction) 1836 .format(input.size(0), target.size(0))) 1837 if dim == 2: -> 1838 ret = torch._C._nn.nll_loss(input, target, weight, _Reduction.get_enum(reduction), ignore_index) 1839 elif dim == 4: 1840 ret = torch._C._nn.nll_loss2d(input, target, weight, _Reduction.get_enum(reduction), ignore_index) RuntimeError: expected scalar type Long but found Float